Competitive Differentiation for Airlines leveraging Social Media Analytics
In the intensely competitive airline industry, carriers engage in a continual struggle to capture and retain the business and enduring loyalty of passengers. Every aspect of an airline’s product, including network structure, pricing, in-flight service and airport presence is designed and delivered with this objective in mind. Product decisions have traditionally been made by combining market research with detailed analysis of data from internal computer systems that handle revenue management, reservations, loyalty, and departure control. Now, with the universal adoption of social media connectivity, airlines have access to a rich source of information about individual customers’ preferences and expectations that can be leveraged to provide a competitive edge.
Taking the perspective of an airline’s customer, we can speculate on what an individual’s expectations might be. Although consumers purchase air travel from an airline, the individual customer’s expectation is much broader. He or she expects a smooth, economical and coordinated journey from door to door, not just airport to airport. The individual also expects to receive updates about potential disruptions to the journey, and swift, informative responses to their own concerns and issues. Airlines have an opportunity to satisfy these expectations and more by tracking and analyzing a passenger’s social media activity. In addition, airlines have the opportunity to earn additional ancillary revenue by positioning offers for products and services based on passengers’ preferences derived from the networks.
Social media analytics (SMA) is a general term that can be applied to a broad range of activities. Initially, the airline must listen to the social channels and interpret and relay the information derived. Airlines have listened to social channels for some time and have frequently derived valuable information to identify trends or defuse negative perceptions. The direct collection, collation and use of the data is the start of the SMA process and generates some value of its own. However, the bulk of the value as well as the competitive advantage of SMA is realized by applying additional components and layers that enable the following types of insights:
- Generation of a deeper understanding of passenger preferences and concerns beyond the superficial collection of data
- Inclusion of any insights about passenger preferences into the overall booking process
- Identification of patterns and linkages among groups of passengers or groups of issues to assess correlation among issues and to unearth root causes
- Development of a response strategy based on the nature of issues raised that includes type of response, response channel, and timing of response
Sense and Respond to Grow Revenue
When planning a journey, the flight is typically the first component completed. This leading position in the sales chain gives the airline a competitive edge in guiding the remainder of the booking journey in such a way as to delight the passenger and earn additional ancillary revenue. The airline can further strengthen its dominant position by deriving intelligence from passenger purchasing decisions.
Beginning with booking, the shopping process can be guided by SMA. Well before a customer considers taking a particular journey, the airline can mine data from social networks and other sources in an effort to create a passenger profile or persona. As part of a persistent background effort to gain a 360o view of the customer, the airline pools data from multiple sources, including its own reservation and loyalty systems as well as from a passenger’s social network (across various platforms) activity to create a data lake, a complex repository of data in its native format – structured or unstructured. From the data lake, valuable insights can be derived regarding the passenger’s preferences, motivations, and buying habits. The analysis may be performed in the data lake, or on data extracted from the data lake, and loaded into other types of data marts. In doing so, the airline creates a context-relevant, unified profile of a customer’s likely behavior and remains poised to capitalize on opportunities as they arise.
Techniques of big data analytics continue to expand and mature. This allows data lakes to provide a flexible, coherent structure for storing the potentially vast amounts of data pertinent to each and every prospective passenger. In particular, the airline can use the information to derive an understanding of the passenger by assessing the passenger’s likely positioning or segmentation in each of three very specific criteria:
- Needs
- Expected behavior
- Value
The segmentation is flexible and fluid, and is dependent upon the context of the passenger’s current activity.
Here, SMA provides the airline a competitive edge by evaluating and scoring the criteria above. The passenger’s needs are understood, their behavior predicted, and their value to the airline computed. Of course, the analytic methodologies are complex, innovative and potentially proprietary. However, many airlines and service providers to the industry have developed tools and frameworks to do this effectively.
Fundamentally, the process is more than a simple reading and review of data collected from individual sources. It relies on identifying and exploiting data patterns across all of the original sources to derive a holistic descriptive mapping of multi-dimensional behavior. With the information, the airline can tailor its interaction with the customer in numerous ways. Doing so provides value to the customer through customized treatment, and to the airline by generating revenue and reinforcing brand image.
Let’s consider the activities of a hypothetical passenger named Bill. Bill is a mid-tier member of Air Possible’s (AP) loyalty program, and flies AP several times a year on business. Bill is diligent about maintaining his profile information on AP’s website, so the airline has knowledge of some important demographic data about him, as well as his booking history. In addition, Bill is active on social media, with a preference for Facebook, Twitter, and TripAdvisor. Although Bill uses different sign-in names on each of these sites, AP utilizes analytic algorithms that provide an 85% certainty of uniquely identifying him on each platform.
For the past few weeks, Bill has been posting about the need for a vacation on Facebook. Given the few vague statements, AP does not yet sense an opportunity to engage with Bill. However, as he accelerates the frequency of his Facebook posts, AP can gauge Bill’s expected behavior more precisely and has raised the likelihood of him booking a trip. Later, AP senses that Bill is researching Key West, Florida in TripAdvisor, and has begun following the Twitter feed of a noted Key West seafood chef. Coupled with his past booking behavior and loyalty status, AP can more accurately assess Bill’s needs and value as a prospective customer, and respond with an approach and offer.
On his next visit to Facebook, Bill receives an invitation to visit AP’s site that specifically mentions deals on travel to Key West. Bill clicks on the invitation and is directed to the AP mobile application with his personal information and destination details pre-filled. He enters his preferred travel dates, receives a list of flight options and select his preference. With the flight selected, Bill is guided to a page of Key West activities to choose from. Upon selecting a fishing trip, he is then offered local transportation options to complete the door to door travel experience. With his shopping completed, Bill is then directed to a payment page with his credit card details available for use. In addition, he is not only given the opportunity to buy, but also the chance to hold the trip for a day. If he chooses this option, he will receive reminders accompanied with additional offers before the hold period expires. Bill decides to buy the trip.
In the period between the purchase and the departure, Bill receives a series of communications containing relevant information and offers. AP sends him details on Key West weather and local events, and also a promotional offer to the seafood restaurant and chef whom he follows on Twitter. Bill is happy with AP’s service and looks forward to his vacation.
In this example, AP utilized SMA to sense Bill’s need and expected behavior, as well as estimate the value of his revenue value to the airline. AP responded by communicating to Bill through his channel of choice, positioning relevant offers and keeping in touch before departure. AP earned revenue that they may have missed if they had not been monitoring Bill’s activity, and Bill’s loyalty to AP is strengthened by the experience.
It’s important to note that airlines must be selective in their use of data and in the communication of their data usage to the passenger. An effective SMA strategy must include procedures to avoid alienating the passenger who may perceive the activities as intrusive or creepy.
Issue Identification and Response to Improve Customer Loyalty and Retain Brand Value
Armed with immediate access to social media, passengers are quick to report positive and negative experiences encountered along their journeys. While airlines are happy to receive the praise of passengers, their criticisms are a source of great concern. Additionally, passengers expect timely, if not immediate responses to their complaints and unmet expectations. With the targeted use of SMA, airlines can now address passenger concerns and retain brand value in a timely fashion.
All businesses have been listening and reacting to social media since the inception of the medium. Airlines, in particular, formed departments of tech-savvy responders to personally monitor the channels and to address concerns quickly. However, such custom response departments rapidly became inundated with the massive volume of social media data available. In addition, without the guidance of SMA, the underlying context, causality and other insights related to the customer’s concern tend to be overlooked. Utilizing SMA as a key component of a rapid, effective response strategy allows an airline to respond to disappointed passengers in a timely manner, construct the response appropriately in terms of context and content, and deliver the response through the appropriate channel. In addition, this can be accomplished with virtually no restriction on the volume of responses required.
In this case, the core of an SMA solution is directed toward understanding a passenger’s true concern beyond the mere collection of data, and responding in a way that would be deemed satisfactory to that passenger as an individual. Although listening to the networks remains the starting point of the process, the SMA tool’s algorithms assess sentiment, classify data into actionable categories, and employ machine learning techniques to identify patterns in data, service and individuals. These results enable the generation of recommended actions based on the context, individual passenger, and historical results.
Let’s consider the example of hypothetical passenger Bill once again. Bill is a frequent flyer on Air Possible, and although generally complimentary of AP’s service, he had an issue today with the lack of AP’s in-flight magazine in his seat pocket. Bill mentioned the problem on Twitter, but was rather neutral in his text. AP contact center agents read the tweet, recognized Bill as a frequent flyer, and also noted that other passengers on the flight had issues with aspects on in-flight preparation: missing magazines, lack of beverage choices, etc. While a reading of Bill’s tweet in isolation might have generated a simple apology, the context provided by the other passengers allowed AP to focus on a training issue at the originating airport. AP provided Bill with a single day lounge pass to his surprise and delight, and the training issue was corrected to prevent future, potentially more critical problems.
In practice, the above steps were taken in a completely automated fashion. The reading and assessment of the data were conducted using advanced SMA algorithms. The analysis was passed to the AP’s CRM system, which selected the resolution and communication mode automatically.
Where We’re Headed
The scope of SMA is expanding rapidly, and airlines have opportunities to capitalize on even greater passenger insights. One current area of rather intensive investigation is image analytics. Users of social media not only post text comments, but photographs of themselves as well. Whether accompanying a text comment, or posted independently, facial expressions and other cues in photos can be assessed to generate insights into emotions and sentiments that can be readily capitalized upon. In addition, certain insights can also be inferred regarding such preferences as vacation activities, hobbies, and interests. Whether deriving positive or negative feelings, an airline can quickly encourage more loyalty by aligning its messaging with assessed interests or fix a problem to appease a detractor.
Beyond “selfies”, users can also post more general graphics that can impact an airline’s brand. Whether it is through a graphic of an aircraft, a logo, an airport, or any other relevant component of an airline’s business, a user’s current opinion of a brand can potentially be understood. For example, a photo of a new shiny aircraft proudly ascending through a bright white cloud against a blue sky clearly indicates a degree of positive brand loyalty. The airline’s CRM system should generate responses to call attention to the post. Similarly, a photo of a dirty cabin or a poorly served meal paint a bleaker picture even in the absence of accompanying text. Here again, the CRM system can spring into action to address the problem and gather further information or offer an accommodation.
Just as passengers are posting photos, some platforms allow the sharing of brief voice messages. Both the content of the message and underlying tone can offer insight into the mindset of the passenger. Tone is crucial, as the actual, verbatim message may be nullified by an assessment of its tone. For example, consider sarcasm. A passenger may post a quick audio note that said, “Boy, did I have a great flight.” On its own, this is a positive message. However, when assessed by a tone analyzer, a high degree of sarcasm may be detected which, especially in conjunction with other available information, should trigger a recovery response instead of a high five for customer satisfaction.
In the airline industry, providing excellent customer service and making your brand stand for something valuable are important differentiators in a time of intense pricing competition. Social media analytics allow carriers to monitor the pulse of their passengers, identify threats and opportunities, and capitalize quickly to improve revenue, enhance customer satisfaction and maintain competitive advantage. As discussed above, the science is expanding rapidly and greater customer insights are available to be revealed from a growing number of sources. Just as importantly, CRM tools and channels are also maturing to enable solutions and responses to the identified patterns.
About the Author
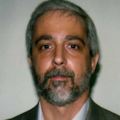